For anyone that follows football on a regular basis, it’s impossible to escape the inevitable cliches around the importance of winning the turnover battle. It’s one of the most popular answers a TV analyst will give when asked to cite a key to victory in a specific matchup. Although there is truth to this statement, this reasoning often lacks key contextual information around when turnovers happen within the game.
The surface-level truth to the often cliche “they gotta win the turnover battle” is shown in the chart below. FBS teams that won the turnover battle won 77% of games overall last season with a win percentage of 71% at +1, rising to 80% at +2 and 88% at +3
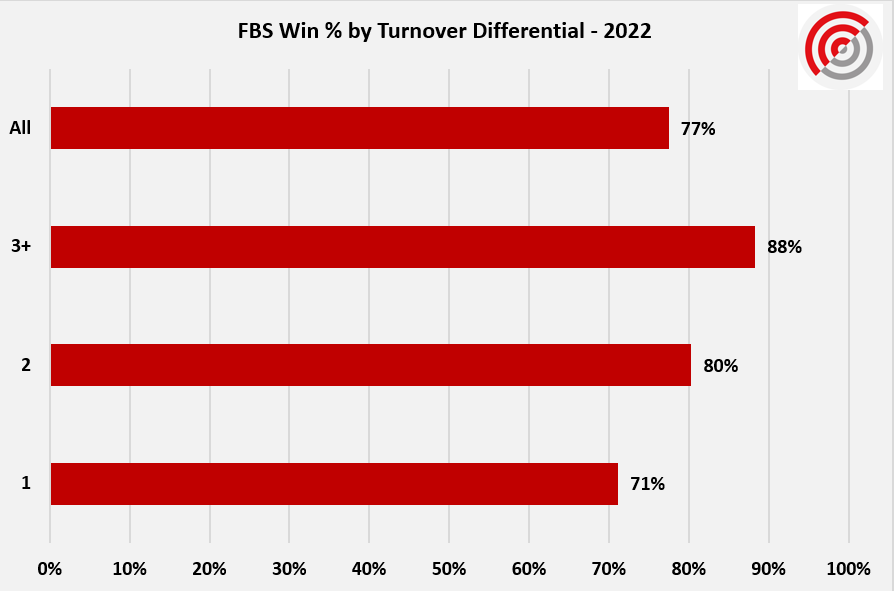
What the chart above does not consider is the contextual in-game factors around when these turnovers occur, specifically that teams playing from behind turned the ball over on about 15% of their possessions last season compared to a turnover rate of 10% for teams playing with a lead or tied. This creates a chicken-or-egg conundrum as it relates to interpreting turnover rates and differentials in football: to what degree are turnovers the cause of a victory in games versus how often are they a mere consequence of the point margin created earlier in the game?
There are several ways to reveal the necessity for contextualizing turnovers on both the team and player level, and this analysis will focus on adjusting INT rates for quarterbacks based on game-specific factors that a raw interception total or rate will not capture.
Adjusting INT Rates for Game Circumstances
In addition to the underlying assumption that turnovers are the definite reason for victory, another common error related to understanding the nature of turnovers can arise in player stats when only evaluating a player’s raw INT % (interceptions / pass attempts). While INT % is a clear improvement on raw interceptions, it does not consider any game-specific circumstances that can influence interception rates, such as Down and Score.
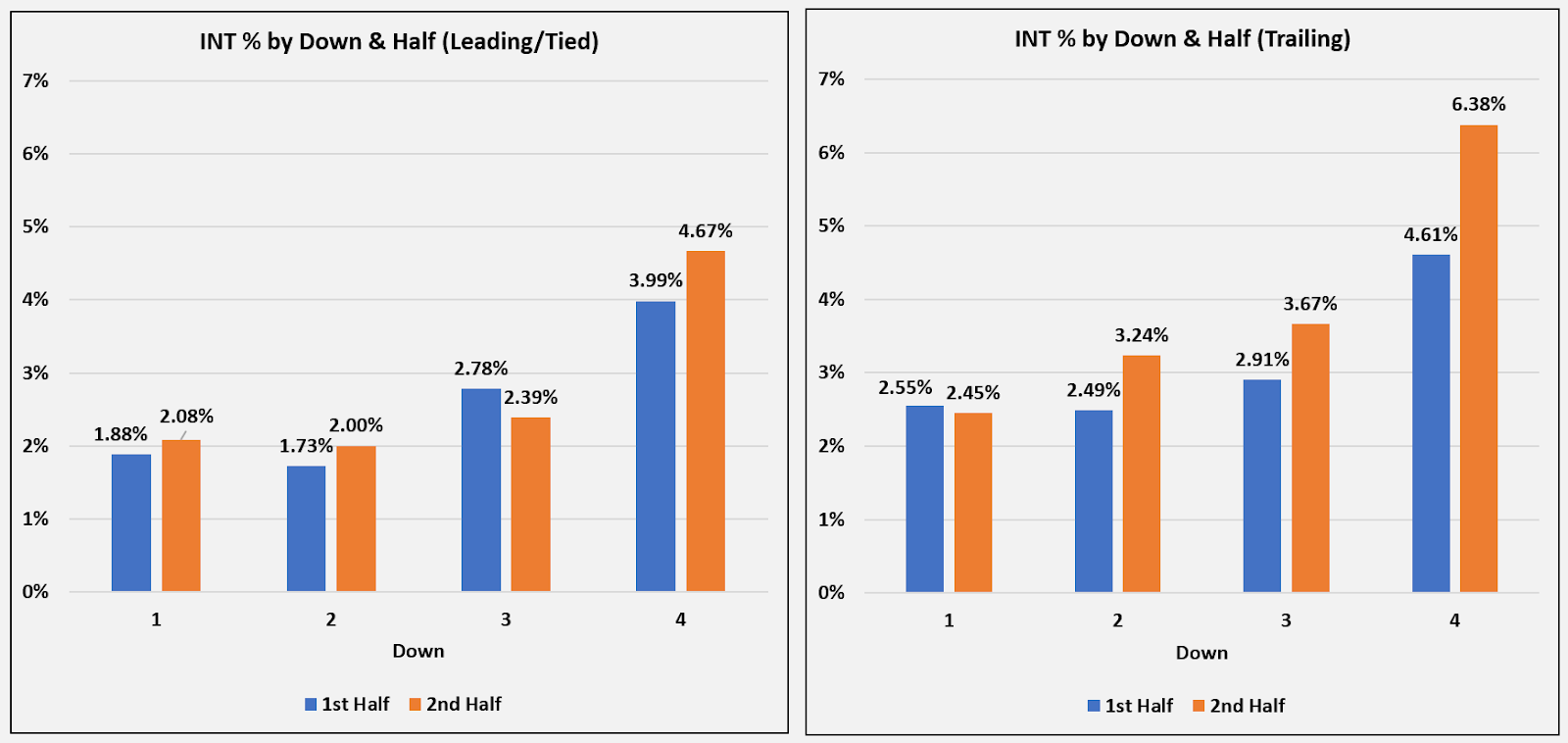
The charts above reveal a number of key trends related to interceptions. The first key takeaway is the noticeable difference in INT % for trailing teams (right chart) compared to teams playing with a lead or tied: interception rates are higher across every down for trailing teams.
Similarly, we see a consistent increase in interception rates down to down, particularly when evaluating the difference between 2nd -> 3rd down and 3rd -> 4th down. The enhanced turnover rate tracks when considering the higher leverage nature of 3rd and 4th down when the defense is close to getting the stop, giving both sides extra reason to be aggressive in pursuing a conversion/stop on the play.
The final takeaway is the noticeable difference in INT rates for trailing teams as the game moves into the 2nd half. As the game progresses, the trailing team will inevitably go on to lose without making up the deficit, giving them more reason to aggressively throw the ball into thick coverage with less concern for how non-scoring possessions end.
Applying Adjustments to FBS QBs
Now that we’ve established that not all pass attempts are equally likely to result in interceptions given the moving dynamics of down, score, and time, we can better contextualize INT rates among QBs by adjusting for these factors. Using the INT rates from the previous charts, we’re able to estimate each player’s interception total from last season based on the down, score, and time factors around each player’s pass attempts from last season.
Because the national INT rate on 3rd down pass attempts when trailing in the 2nd half was 3.67% last season, we can establish an expected interceptions level (or xINT) for this kind of pass attempt to lead to 0.0367 interceptions. Aggregated over the course of a full season, we can see how many interceptions we expected a QB to have thrown based on both the volume and circumstances around his pass attempts (xINT). We can then compare the QB’s actual INT % to his xINT % to generate an INT % versus expectation, shown in the far right column of the table below.
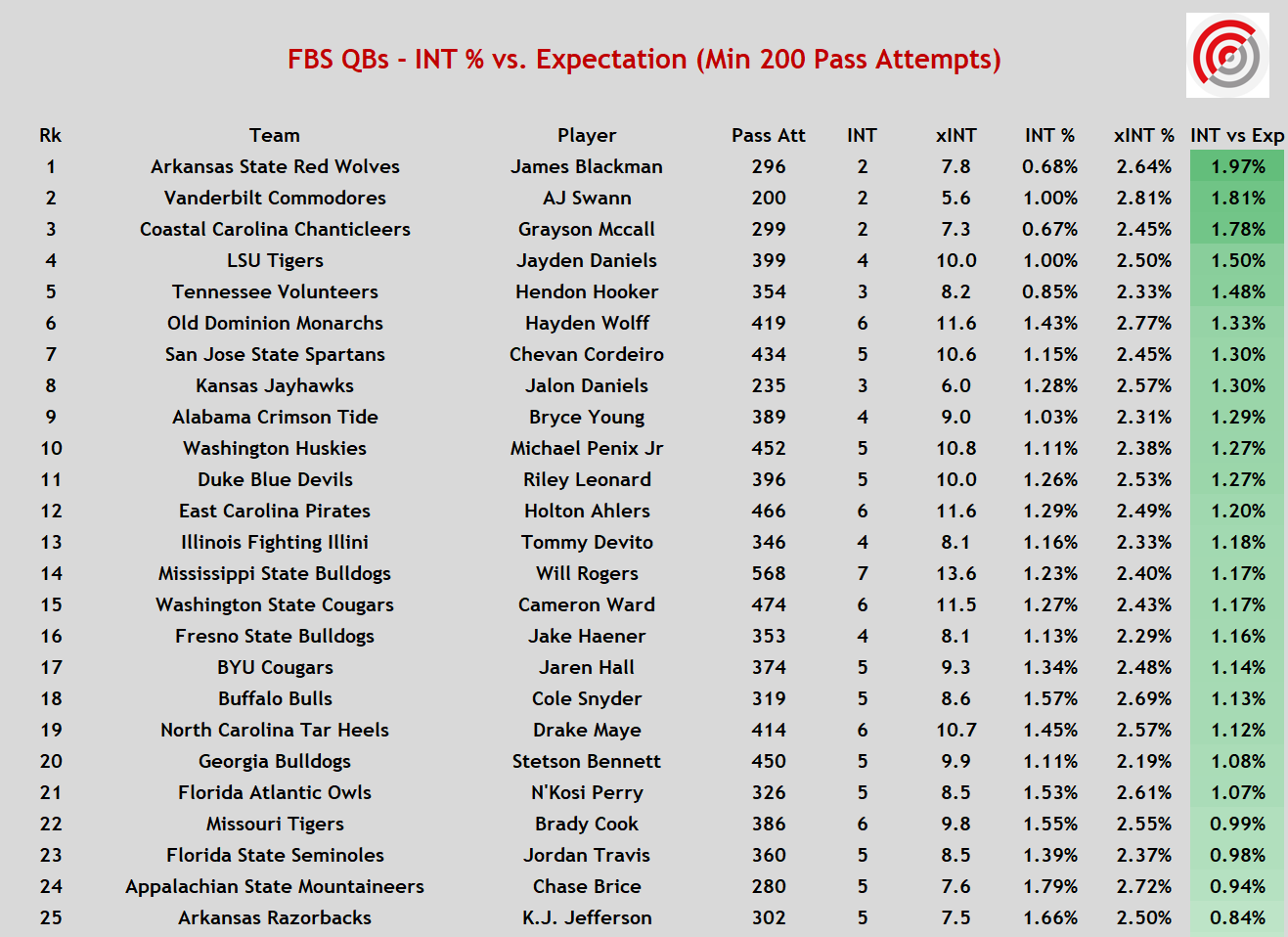
Having adjusted for game circumstances, the chart above gives us a good idea of which quarterbacks were most effective at avoiding interceptions last season. Several noteworthy names appear on the list, including NFL draftees Hendon Hooker, Bryce Young, Jake Haener, and Stetson Bennett, in addition to some of the better returning QBs in college football like Michael Penix Jr, Drake Maye, and Jordan Travis.
Stetson Bennett is of particular note - his xINT % of 2.19% is easily the lowest expected INT rate for any player in the chart, illustrating that his Georgia Bulldogs were frequently playing with big leads, which meant that Bennett wasn’t forced to make many risky throws in a comeback effort. Bennett sported one of the very best raw INT rates in the country at 1.11%, allowing him to crack the top 25 in INT % versus expectation despite not attempting many passes in risky circumstances.
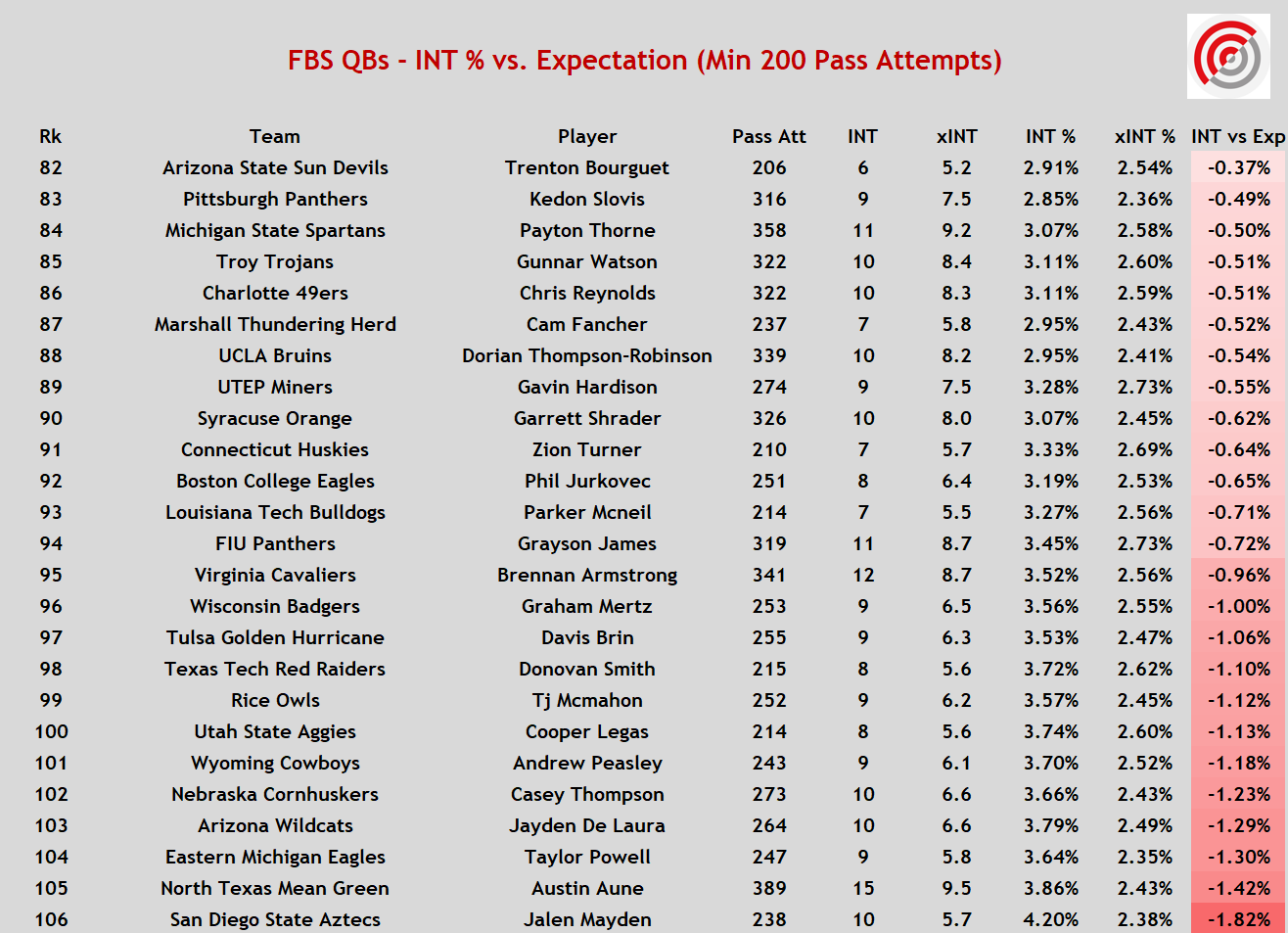
The bottom of the list reveals the 25 quarterbacks with the largest negative discrepancies between their raw INT % and XINT % last season. UCLA’s Dorian Thompson-Robinson, a fifth-round selection of the Cleveland Browns, was one of the most productive QBs in all of college football last season, though his presence towards the bottom of this list means he threw more interceptions than average given the circumstances around his pass attempts.
Conclusion and Further Application
The simple process of adjusting interception rates for down, score, and time remaining reveals the shortcomings of raw turnover metrics without consideration towards game factors that heavily influence when and why turnovers tend to occur. While general football media may continue talking about turnovers in overgeneralized cliches that don’t accurately reflect the cause/effect dynamic of turnovers, those that explore the concept of turnovers with data are likely to reach very different conclusions about how turnovers should be interpreted in football.
Mike Bursik
AMF Product Lead
@MikeBursik