This article first appeared on iGB America on 19 May 2022.
Small edges can make a big difference in soccer trading, yet, as StatsBomb Chief Marketing Officer Simon Banoub explains, many businesses still rely on incomplete data for their models. Could improved versions of tools such as expected goals provide sportsbooks with a much-needed edge?
Soccer trading is a mature market with sophisticated global players. The edges are razor thin, and competition is fierce to land skilled modellers capable of creating margin. In that environment, how can a sportsbook differentiate itself? Could better data be the answer?
The data that sportsbooks purchase is pushed through algorithms to create predictive models such as Expected Goals (xG). But most of the xG models on the market have massive limitations, forced upon them at the input level by data providers who have, for one reason or another, failed to innovate their offering. And if these models are built upon incomplete data, it stands to reason that their predictive power is missing something as well.
To give an example of these limitations, take the most high profile goal of last season, in one of the most traded games of the year: Kai Havertz’s winner for Chelsea against Manchester City in the Champions League final. Havertz latched onto a through ball from Mason Mount, rounded the onrushing Ederson and calmly rolled the ball into the unguarded goal from 12 yards out.
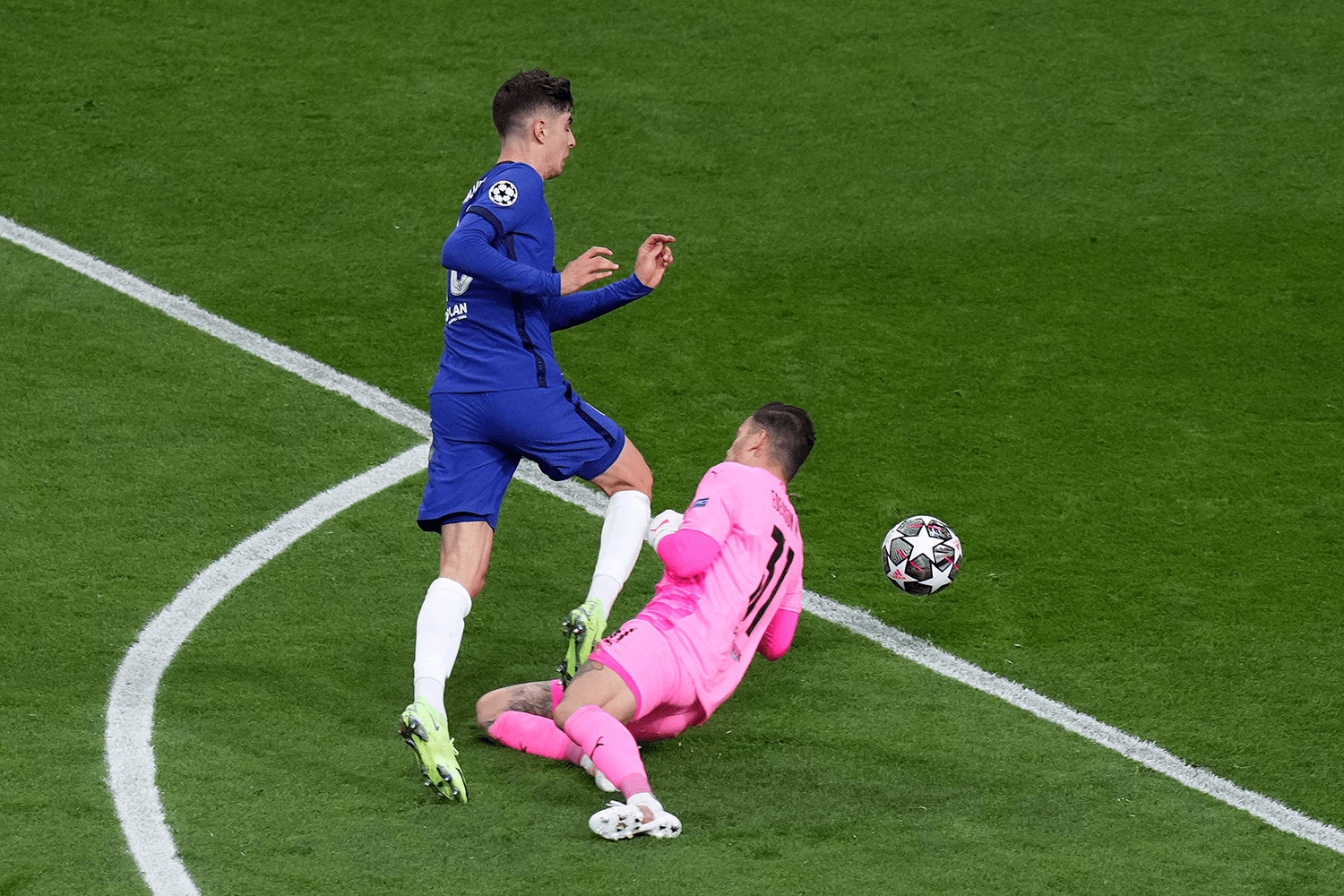
To everyone watching this game, the final finish was an easy one. But not so to a traditionally available data feed, shorn of vital information regarding the lack of defenders, the bypassed goalkeeper and the gaping net. The most commonly used xG model rated this goal at 0.36, meaning that it gave Havertz only a 36% chance of scoring. That fails the eye test for anyone who has watched or played the game.
This is where more advanced data comes into its own. Over the last few years, upstart companies have created enhanced data with extra information around every action on the pitch, providing key additional context.
For critical events like shots on goal, this includes the position and state (as in, whether set, moving or grounded) of the goalkeeper, the position of all defenders, and the height of the ball at the moment it is struck. In the case of the Havertz goal against Manchester City, those factors help elevate the chance from an xG of 0.36 to an xG of 0.81 according to the StatsBomb model – a much more reasonable 81% chance of scoring.
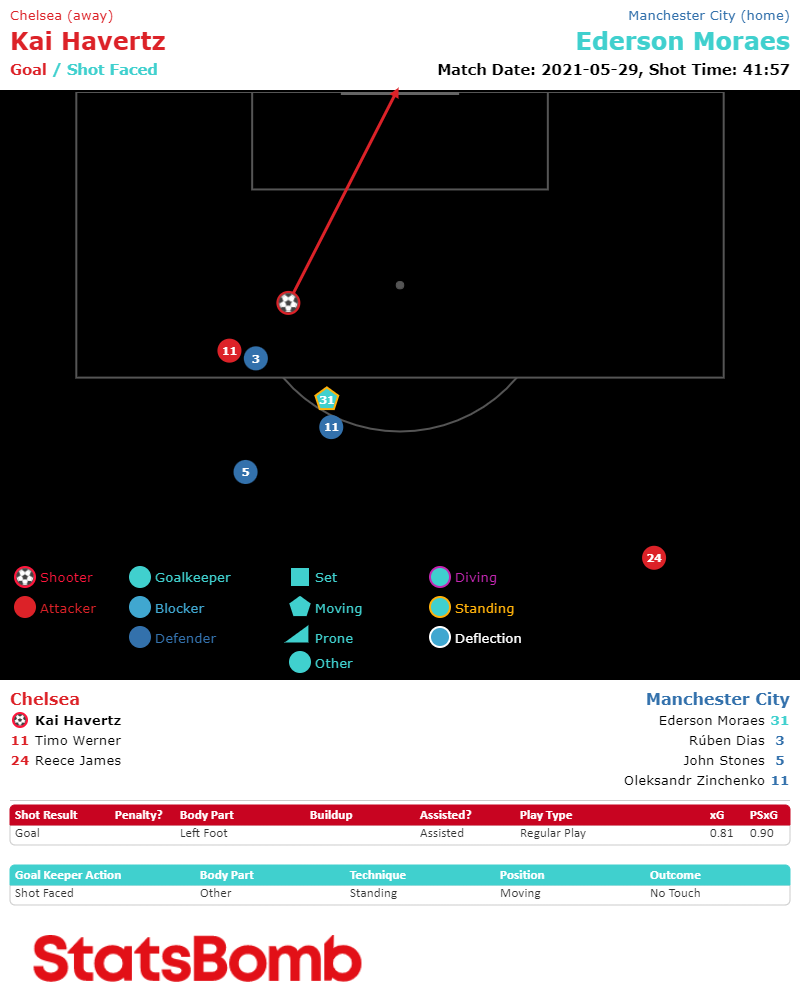
If trading teams extrapolate a difference like that across all of the goals in thousands of matches in hundreds of competitions around the world, the chance to gain an edge becomes apparent.
Shots into an empty net or from positions behind a defensive line represent the most obvious examples of the benefits of a model that takes into account the position of the goalkeeper and defenders, but this additional information also serves to increase the accuracy of xG values in the opposite case: when their combined positions actually reduce the possibility of a goal being scored.
Take, for example, the early February Premier League game between West Ham and Watford. In the first half, Saïd Benrahma took a shot from the right inside the six-yard box. The most widely used xG model took into account that it was a close-range shot and despite a relatively acute angle to goal assigned it an xG value of 0.42. But a newer model, taking into account the proximity of the goalkeeper shutting down the majority of the goal and the covering defender on the goal line, gave the chance a more realistic xG value of 0.15.
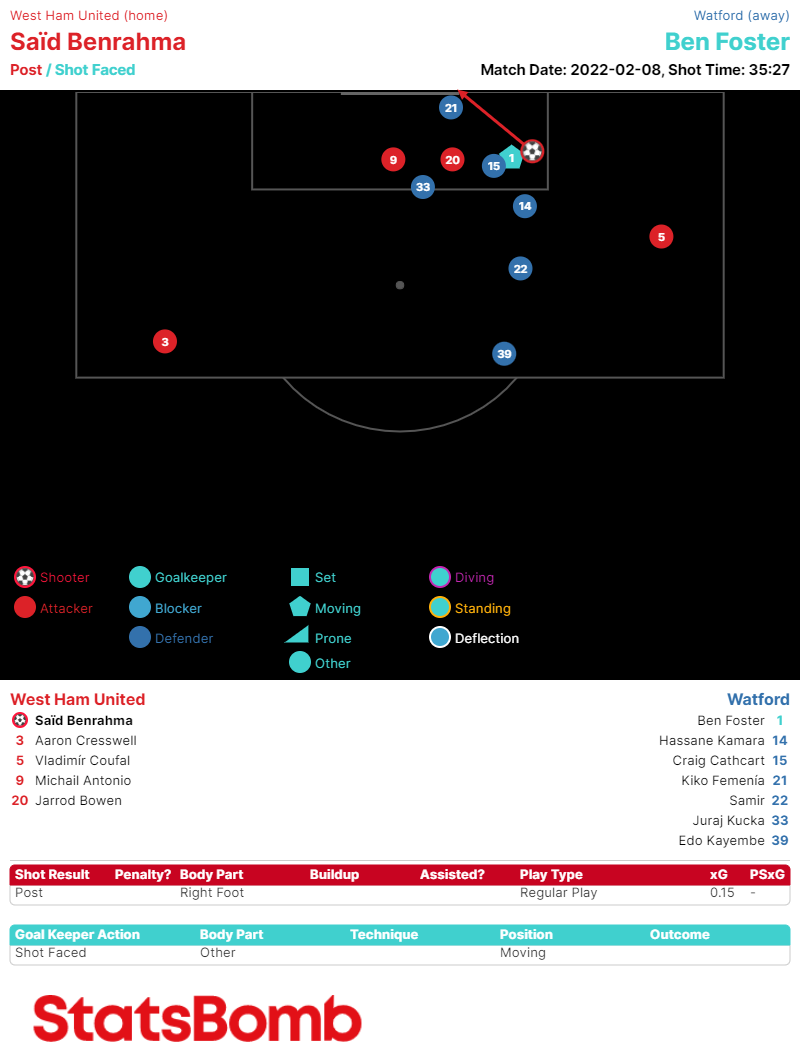
The height of the ball at the moment of impact is another factor that is generally a very subtle differentiator but that does provide more realistic values in edge cases where it has a genuine impact on the ability of the player to finish a given chance. Situations like awkward volleys with the ball above waist height make it much more difficult to score, while the difference between firing a grounded ball into an empty net versus one that’s bouncing up off the turf can turn an easy chance into a more challenging one.
In aggregate, the inclusion of all this extra information means that newer models produce a much more natural curve of xG values than traditional ones that rely on a collector-controlled tag to identify and artificially bump up the value of chances deemed to be of good quality.
Better input data is especially important in the case of a predictive metric such as xG but the greater granularity of the more modern data feeds now available also adds more contextual information to all aspects of performance. For instance, there is now the ability to understand the role that pressure plays in all areas of the pitch, and how different players adjust their game accordingly.
Surely the time has come for the battleground to move from getting the smartest people through the door to giving those smart people the best possible tools to work with. In a world where small percentage gains make a big difference, overlooking the chance to optimise critical data inputs means that sportsbooks could be missing out on an edge. And at what cost?
If you're a sports trading organisation and want to talk to us about how StatsBomb data can enhance your edge over the market, get in touch today.