As a coach of my 11-year-old son’s 9-a-side team, nothing frustrates me more (except for kids taking a pair of scissors to their socks – but they wouldn’t let me write a blog piece about that) than players not getting their head up, taking too long on the ball and failing to pick out a pass to a teammate in open space.
It kills the attack, our team invariably loses possession, little Tommy from the end of the street has one ricochet off both hands, the referee, who is also the opposition’s coach, plays on, and we ultimately go on to lose 7-4.
I’m being slightly facetious, but as a basic principle, moving the ball quickly and accurately, to teammates in space, is fundamental to the way that a lot of elite teams now play. If you don’t have players in your team that can do it, then you lose the high rewards that your high-risk strategy of playing out from the back and baiting the opposition yields.
Think about De Zerbi’s Brighton knocking it about deep in their own defensive third, baiting the opposition. It amounts to nothing if they can’t move the ball forwards quickly and accurately when they manufacture an opportunity to do so.
But of course, passing the ball quickly, accurately and into more advanced areas of the pitch is not an easy thing to do. Players that can do so reliably and frequently aren’t easy to come by. Which got me thinking…. how can we use data to identify these types of players?
So what do we want?
You could make an argument for this being a good trait for players in all positions to have, but I think most would agree that in the midfield is where it can be most impactful, and for the purposes of this piece I’m going to focus on midfielders only - I’ve included wide and central midfielders, but if you’re looking specifically for a wide midfielder then you may want to discount those in the middle of the park as this process will naturally favour them.
So let’s clarify the qualities that we want in this type of midfielder. We’re looking for a player who:
- Is heavily involved in their team’s in-possession game
- Can execute a quick pass (first time pass or otherwise)
- Can spot a teammate in space
- Can directly improve our team’s chances of winning football matches
There are other traits you could make a valid argument for including, but in the interest of keeping this reasonably succinct, let’s stick with the above. Now that we have a clear set of characteristics, we can set about using data to get a proxy for identifying our midfielder.
Heavily involved in their team’s in-possession game
How can we identify midfielders that are instrumental to their team’s play? One way is to look for midfielders that play a lot of passes. But because I don’t want to end up just curating a list of midfielders who play in high possession teams, I’m calculating a ratio of each player’s passes attempted relative to their team’s total passes.
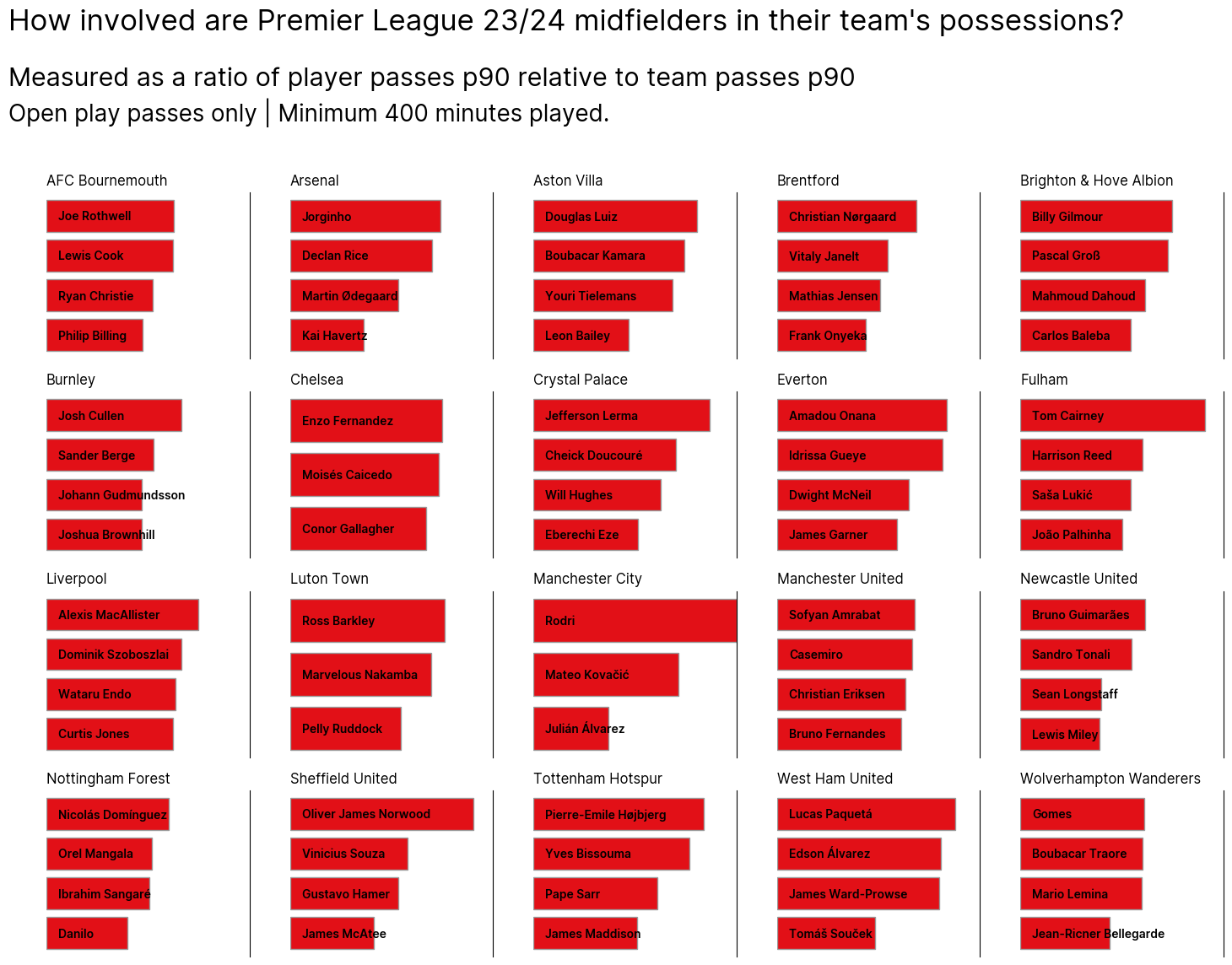
Although this is a fairly simplistic calculation of a player’s total passes p90 divided by the team’s total passes p90, and it doesn’t account for the differences between when the named player is on or off the pitch, it passes the eye test for what I’d expect for most teams.
Rodri is pivotal in Manchester City’s play, Jorginho and Declan Rice pull the strings for Arsenal and there’s a more even split of responsibilities at Liverpool.
Can execute a quick pass
Now for the fun (admittedly, depending on your definition) part. I’m going to use the timestamps in our event data to get a proxy towards players that move the ball quickly. Almost all passes – and certainly the ones relative to what we’re trying to identify here – are preceded by a recorded Ball Receipt in StatsBomb’s event data. Therefore, by calculating the time between a ball receipt and the subsequent pass being made, we can see how quickly a player moves the ball on.
A time difference of 0.0s would indicate first time pass. I’m going to widen the net a little to a slightly arbitrary 1.0s. This will obviously rule out some ‘quick passes’ that are, say 1.1s, but you’ve got to draw the line somewhere, and if you’re doing this yourself you can play around and adjust the threshold.
I’ve also filtered to look at passes made with the feet only. Headed passes will, by their very nature, nearly always be first-time passes in this definition, and that’s clearly not something that I’m interested in.
So with quick passes identified, we can now calculate the completion rate of these passes and compare them to xPass values. This will give us an idea of whether players are completing these types of passes more often than they are expected to do so on average.
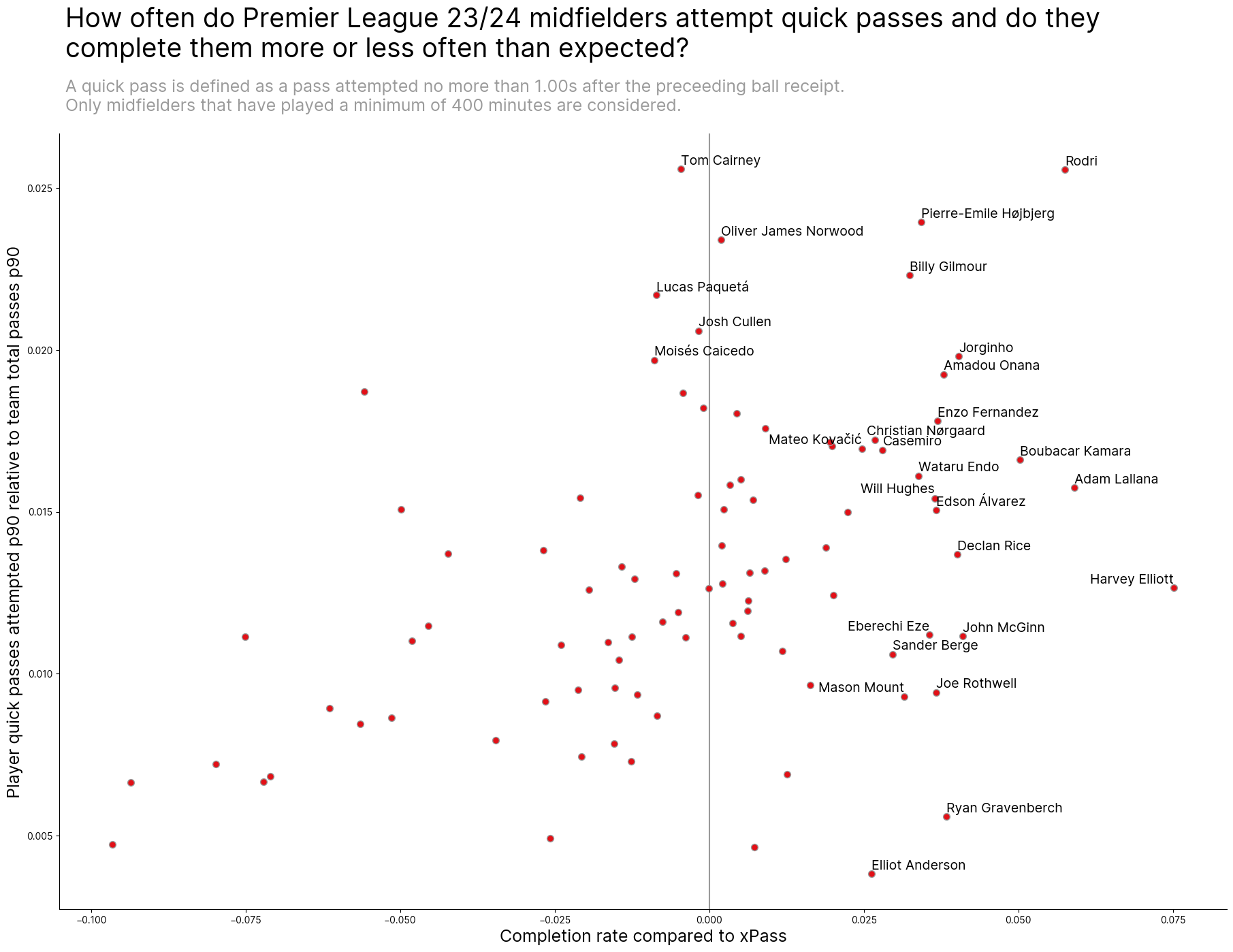
So not only does Rodri complete a high % share of his team’s passes, he also has a high rate of our definition of quick passes, and completes these passes at a notably better than expected rate. Decent player.
Harvey Elliott is the only midfielder in the Premier League (minimum 400 minutes played) that is completing these passes at a higher % above expectation, but he is attempting them at around half the rate of which Rodri is. Doffs of the cap to Højberg, Jorginho and Billy Gilmour who are all attempting a high volume of quick passes and completing them at a higher rate than xPass suggests that the average player would.
Improves their team’s chances of winning
Undoubtedly there is value, on occasion, in making a negative quick pass that moves the opposition and allows for a positive pass further down the possession chain. And with a bit of work, you can also identify players that contribute in this way using event data. However, for the purpose of this exercise, I’m going to look for players that directly have a net positive impact on their team’s chances of scoring with their quick passes. To do that I’m now going to look at the OBV of the quick passes that I’ve identified.
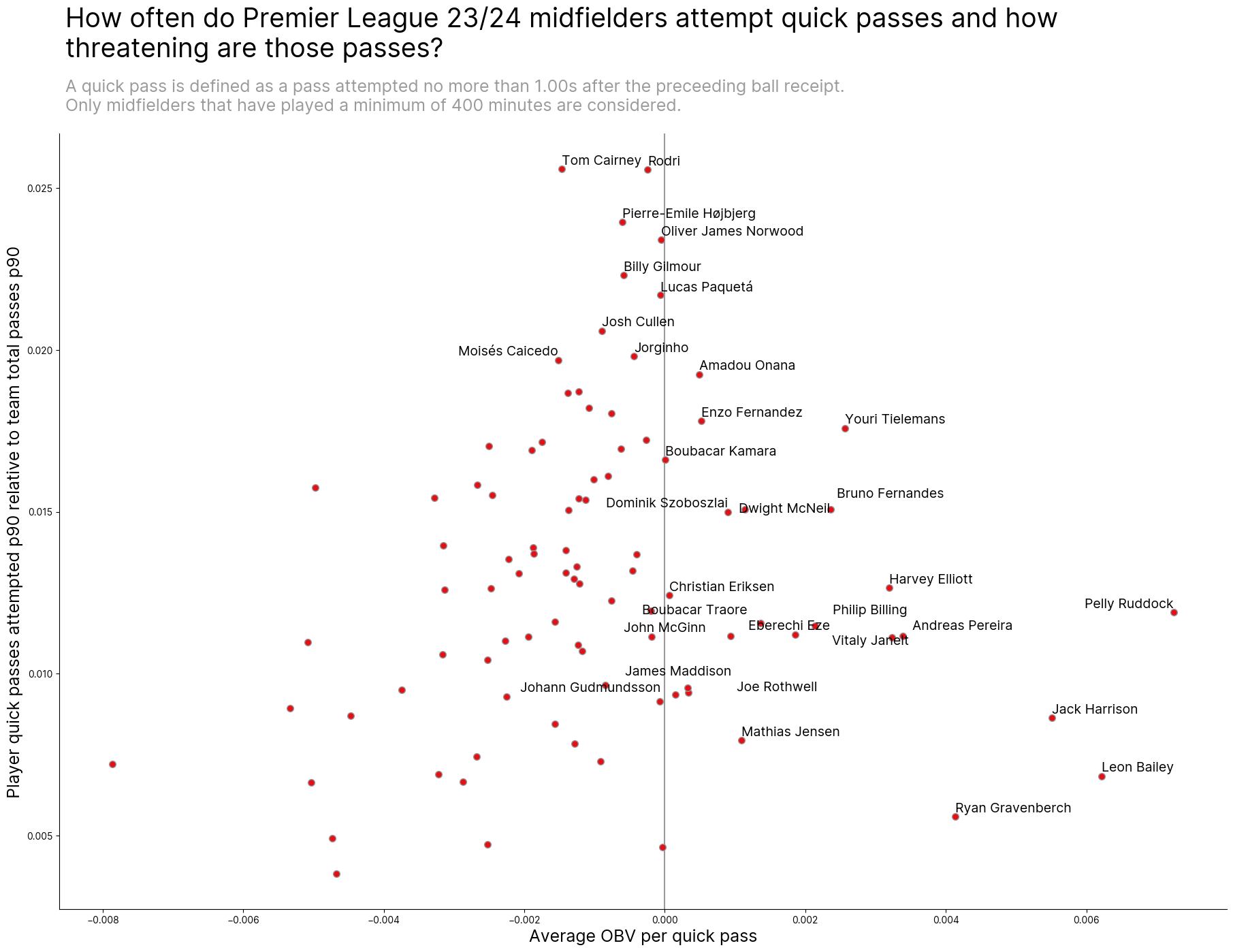
It’s quite clear that the players who are making more of these quick passes are doing so at a fairly neutral threat rate, on average. Rodri, Højberg, Oli Norwood, Gilmour, Lucas Paquetá are all hovering around the 0.00 line for OBV per pass, and there’s a general trend of gravitating towards that line as the volume of passes increases - perhaps a nod to this being a difficult skill to excel in.
Shout out to Pelly-Ruddock Mpanzu, Jack Harrison and Leon Bailey for being fairly potent when they do play quick passes. Harvey Elliott also stands out with a reasonably high rate of execution and a strong OBV per pass value - if Liverpool can get him involved more often then he could be a valuable resource for them this season.
Finds teammates in space
The final piece of my quick-passing-midfielder jigsaw is an ability to find teammates in space. For this we can dip into the 360 data set and look at which of the quick passes that we’ve identified land at a teammate in space.
Here I’ve chosen to look at passes received in a space of 5m or more – again, this is a fairly arbitrary amount and you can adjust as required. I should also point out that I’m not adjusting for opportunity here, this only looks at the frequency of which a player finds a teammate in space, there is no accounting for the number of times that option is available to them – if your teammates don’t find space, then you can’t be expected to deliver the ball to them in space.
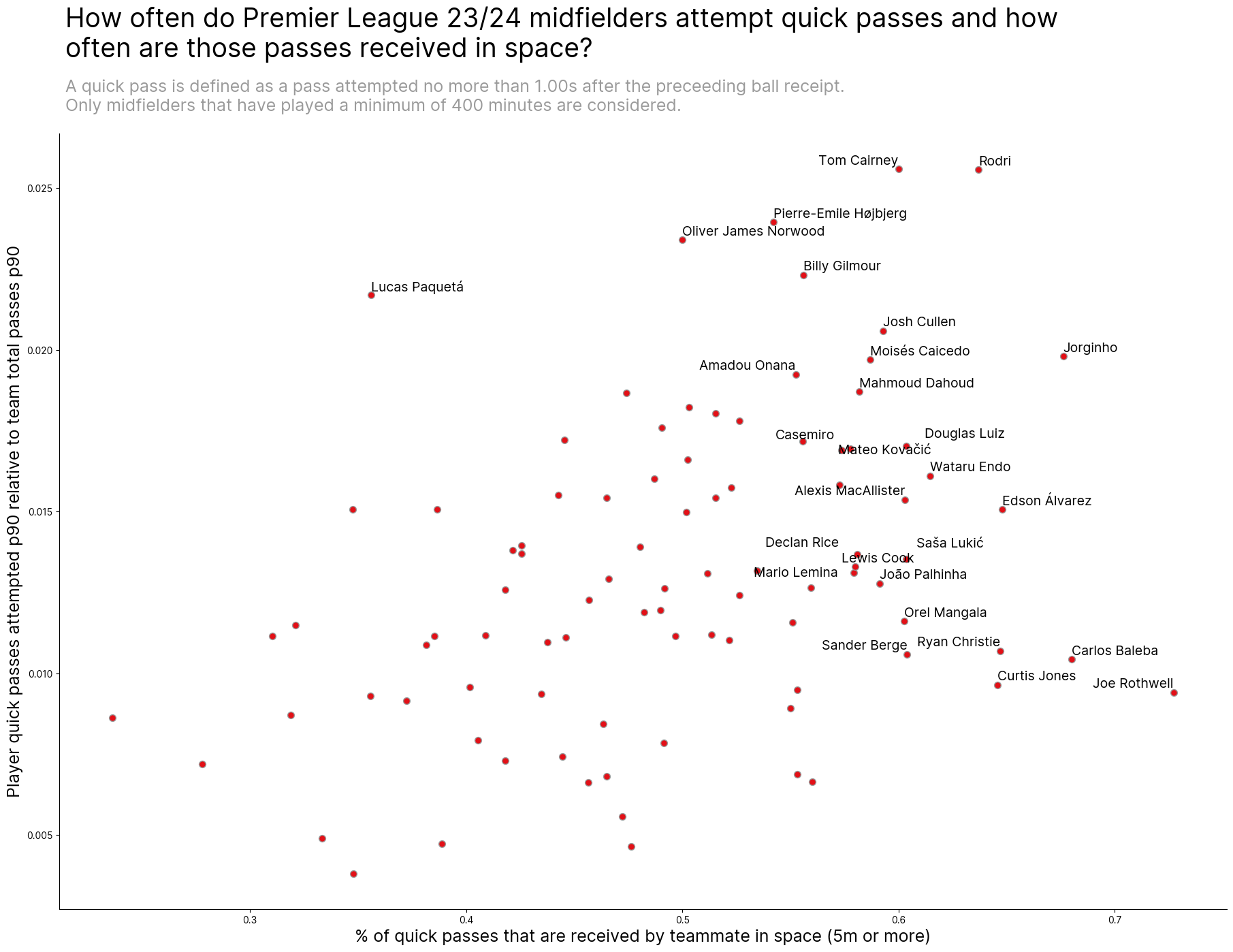
This Rodri guy seems to be doing a lot of things right... might want to take a closer look at him.
Tom Cairney may be completing quick passes at a lower rate than expected and with a negative average OBV value, but he is at least finding teammates in space with a high percentage of those passes.
What does all this mean?
To conclude, if you want to improve your team’s midfield, go and sign Rodri or Jorginho…. But if they don’t happen to be within your budget, you can apply the same logic to other leagues around the world, say Japan’s J1 League, the Scottish Premiership, or Czech Fortuna Liga, for example. Apply a few filters on minimum requirements for some of your new metrics and see what it shakes out:



Caveats
Of course the above is not exhaustive, there are plenty other factors you might want to throw into the mix. There’s a very good argument to be made for adding press resistance as a criteria - although that is baked into the xPass model somewhat. Game state is also a potential component that you’d want to consider – the way that a player passes the ball can differ notably when winning or losing. Pass length, pass direction, pass height and pass start/end location are all also things that you might want to, and can, assess.
Ultimately, you can shape this how you want, to fit the criterias that your team cares about. And whatever way you mould it, it’s not going to be a silver bullet to finding you the player that you want or need. But it absolutely can be another extremely useful data point that helps to build a clearer picture and point you towards a more curated shortlist of players to scout and assess in more detail.
One thing’s for sure, when the Dundee District Youth Football Association finally cave to my lobbying and bring in StatsBomb as their official data provider of the under-12s league, I know what metrics I’ll be looking at to find our next deep-lying playmaker.
