For years, we’ve used percentage of passes completed as an evaluation tool for how good a passer a player is. The problem is that basic passing percentages are meaningless for player evaluation.
This creates a difficult situation for clubs looking to recruit excellent passers from a data perspective, especially since football is a passing game that just happens to have goals at the end of it.
Think about it… Your average football match features 3 or fewer goals.
And about 25 shots.
And around 1000 passes.
And yet we have very little information about the quality of a player’s passing, especially outside the final third.
Here at SBS we think about passes in the same way that everyone now thinks about shots and expected goals (xG).
A team can pass between their centerbacks all day long against no pressure and put up big numbers and high percentages, but that doesn’t tell you anything. Prior to now, passing ability among players was actually one of the most difficult things to assess statistically, so teams were forced to rely on scouts for this information.
Our new model changes all of that.
The Basics
- Long passes are harder to complete than short ones
- Vertical passes, especially in the opposition half, are harder to complete than most horizontal ones, though this rule gets a bit squishy around the box.
- Passes from outside areas to central areas are harder to complete
- Passes as a whole become far more difficult to complete as you approach the goal.
“Duh… this is obvious.”
Alright smart guy...
How Do You Quantify It?
To better assess player passing ability, we have taken a two-stage approach. The first thing we have done is to take 20 million open play passes from the Opta data set and create baselines for all passes attempted from one location to another location on the pitch. We include factors like time, distance, where on the pitch it occurred, the type of pass, player position, and a few other elements we think are important to produce a general pass expectancy.
The second stage of the model then adds in the players. How difficult are the passes they attempt? Do they complete them more or less than expected? We then take that information and roll it into a rating
The explanation is simple, but the data work and computational power required to crunch all of this information is massive.
History
We’re not the first analysts to think about doing this. Back in 2012, the current Head of Analytics at Toronto FC (Devin Pleuler) produced a piece on the main MLS site raising the bar on how we evaluate player passing.
The first active model that I knew about was produced by Łukasz Szczepański at Brentford/Smartodds. I had this idea that passing could be evaluated differently and mentioned it to Phil Giles one day in 2014, and he said something like, “Go talk to Lukasz, that was his thesis topic.” My brilliant, new, “original” idea had already been worked on for years by someone else. Welcome to sports analytics, kid.
Looking backwards through transfers and players they have been linked with, it seems fairly likely that Arsenal and StatDNA have had something like this active for years. Jaeson, Sarah, Fran and the crew are all incredibly bright, and this is a moderately common theme that most football analysts will twig upon naturally in their learning journey...
And then not talk about publicly ever again.
We operate a bit differently. I’m vocal about wanting to continue moving football stats forward, and part of the reason StatsBomb exists is to do exactly that. StatsBomb Services is a separate entity, but in my head it shares a similar mission. I continue to write about what I have learned over the years inside and outside of football. While we’ve been creating a lot of IP that is only available in private, Passing Ability score is a big enough deal that I wanted to bring it out front and say, “Hey, we think this is really important and have for years, but now it’s time to talk about it.”
Paul Riley has been publicly discussing his work in this area since the winter, and deserves credit for taking some computationally heavy modelling work and simplifying it to where it’s easily understood and possible to replicate in other places.
Implementation
This information is currently widely available to customers on the StatsBomb IQ platform, and as an extension it’s also available to our fans via radars we tweet. Since passing accuracy doesn’t really deliver useful information, Passing Ability score has been swapped in on those radar spokes, and it’s being listed as a percentile rank in the population.
Toni Kroos is 100 – the very best rated passer out of 22000 in the data set we have, while Romelu Lukaku is 0.16 (or one of the worst).
It takes about 1000 passes before scores begin to stabilise, so be aware that players with smaller sample sizes may see more change over time than established players.
In addition to the percentile rank, we are going to gradually release more passing profiles for players and teams throughout the next year. I don’t want to say too much as this is still in the design phase, but our aim here will be to offer clarity in the most important and frequent tendencies at both the player and team level, and to add easy to interpret visualisations for club personnel.
I don't see this discussed very often, but when recruiting players for football, you do not care about single number evaluators. What you really care about are skill sets. Passing Ability rating adds new information in an area that hasn’t been widely available previously. However, this is just the beginning of where I want to take this analysis.
There are certain types of passes that are more valuable than others, and some are requirements of specific roles in tactical systems. What if you could sift through the data and find guys who can make those passes? What if we made that easy for you? What if I could show you detailed information for every pass that a player makes from different areas he’s standing on the pitch AND show you whether he’s good at those types of passes or not? That’s where we’re headed.
Below is a list of the top 5 active players per position in Passing Ability in the Big 5 Leagues

This largely passes the smell test. It also flags up Tom Cairney as one of the best passing midfielders in England right now (not listed above because he plays in a lower league). Can Fulham hang on to him for another run at promotion?
What’s fascinating is that you also get very clear bands when you separate guys by position just looking at average difficulty of pass. This graphic is massive and needs a big screen to tuck in (sorry mobile users!), but a couple of points about it below.
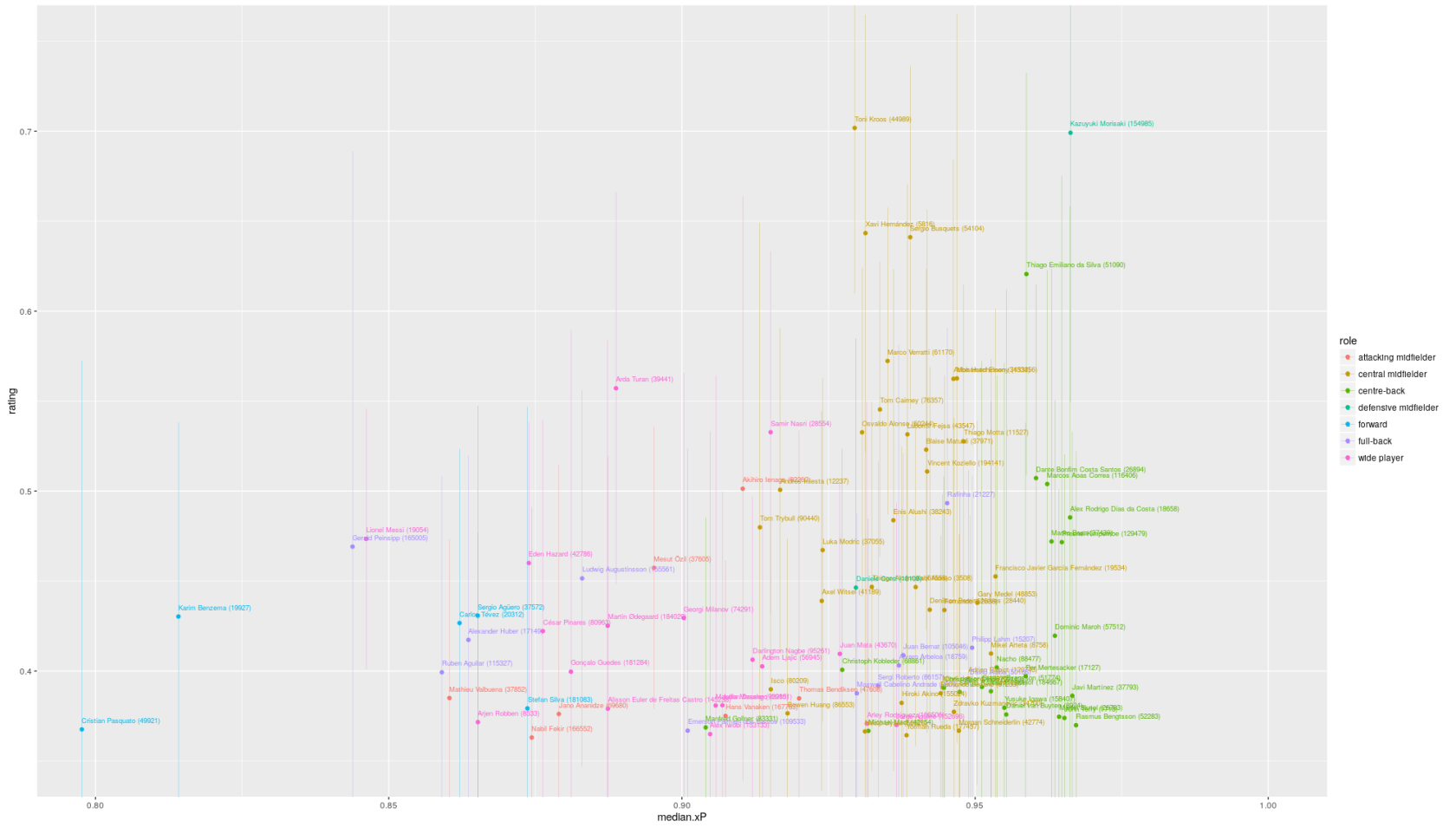
- The average difficulty of passes attempted and completed by Messi is insane (he's the pink dot to the far left), especially when you consider the volume. It’s yet another example in an endless stream of how completely unique he is as a player.
- Ruben Aguilar is on here as one of the best passing fullbacks. I quietly flagged him to a couple of friendly teams two months back. He moved on a free to Montpellier.
- Ludwig Augustinsson is also on here and has also already moved this summer. As I mentioned on Twitter, I’ve been a fan of his for pretty much his entire time at Copenhagen.
How Should You Use It?
It’s just another data point in the player evaluation process. Possession heavy teams may want to weight this a bit more than normal, especially in midfield, but there are plenty of notable players that evaluate as fairly poor passers who excel in other areas. (Romelu Lukaku and Shane Long among them.)
On the other hand, look at Real Madrid, who have won the Champions League three out of the last four seasons. Midfielders Toni Kroos and Luka Modric are in the top 30 players in overall passing ability out of 22,000 players in the data set. There might be some value in packing your squad with as many elite passers as you can afford and letting them run the show.
From a practical perspective, I thought this was one of the most valuable tools we had available to us when recruiting players for Brentford and Midtjylland. We didn’t always let the model numbers rule our evaluations, but it was certainly a useful guideline, and helped us better rank the targets that we wanted to recruit.
How Can This Be Improved?
It’s important to remember, what we’ve produced is a model and not a perfect reflection of reality. There are a couple of players that I would personally quibble with, but on the whole, the vast majority of ratings definitely pass the smell test.
However, tracking data would make a huge difference on how we evaluate both the baseline expected passing numbers and how we evaluate players from an ability and decision-making process. (At the moment these two things are baked into the same cake.)
On the flip side, I fear you’ll need a couple more years of hardware development to start coping with tracking data, or your model fitting time might be measured in years and your club will need to be owned by a billionaire just to pay your AWS bill.
What’s interesting is that I’ve seen outputs from a couple different types of xP models now and they all have various bits you can quibble with. One model I saw probably didn’t penalise players who constantly chose easier options quite enough when it came to the final rating. Others likely don’t credit players who succeed at pulling off truly valuable chance creation enough – the type of players that are high risk, but high reward in their passing. Thankfully, we have other metrics that we use to help in those areas.
We could also use more data. As I mentioned above, we have about 20M open play passes we have crunched to end up here, but one of the issues is that we have a real breadth of information (30 leagues), but not nearly as much depth as I would like (only 4 seasons). More data throughout player lifespans would teach us about how this ability develops as players age, and how much time can affect rating volatility.
What’s fascinating to me now is that there is so much information here and so many ways to use it that almost no two independent passing models will ever be alike. People think different expected goals models is an oddity, but as more people/companies develop their own, you simply will end up with different passing ability ratings from different models.
This one is ours, and though I know we’ll improve it in future iterations as we do everything, I’m already using it in player evaluation and recruitment.
How Does This Impact Fans?
I’m writing this post is because I got a host of questions about it when I was testing radar output last week.
“What is this rating?”
“Why bother?”
“It’s all a bit Squawka, isn’t it?” *blocked*
One of the reasons I often trial run things on Twitter is because it flags up problems very quickly. The first problem that I knew was an issue but hadn’t resolved was the ratings output. It was just very abstract. A German friend of mine saw the new output and said, “Why don’t you use percentiles?”
*lightbulb*
*head desk*
OF COURSE we should just use percentiles for this completely unapproachable model output number, why am I so stupid? So we did that, and then we adjusted the boundaries for positional cutoffs again, and here we are.
Beyond that, this is probably the start of where football analytics moves beyond what the average fan can reproduce with any fidelity. Thankfully, it’s still easy to understand and use. Whether it will be widely available information is another question entirely. As much as I would like to take what we’ve built for StatsBomb IQ and make it available to fans, the data license for doing so would be exorbitant. I could, however, see a league doing this type of thing on their own site as a fan engagement project – YO, PREMIER LEAGUE… call me - but that’s about the only way it’s likely to be financially feasible in the near future.
In the meantime, hopefully you now understand why I think passing completion percentages are pretty useless for players, where our Passing Ability ratings come from and why I think they matter.
Thanks for listening!
