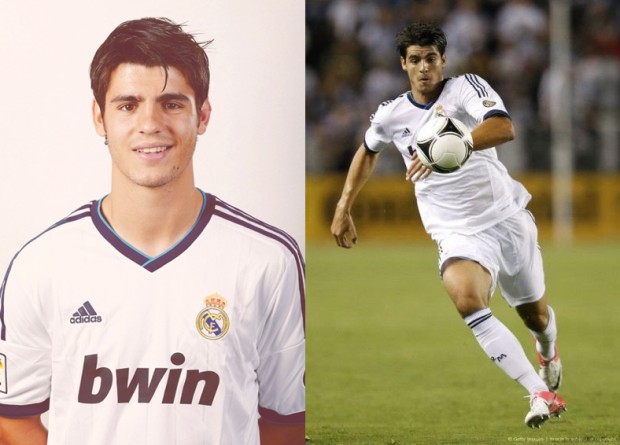
If you listened to the podcast yesterday, you know that there’s one guy that I tabbed as the best attacking prospect in Europe. Ben expressed a fairly strong degree of scepticism on Twitter when I initially said this and then again on the pod, and rightly so.
Young player scouting and prediction is basically impossible. When you do it via the eye test and someone doesn’t work out, you shrug and point to transfer numbers that say 50% of ALL transfers fail. Guys get injured. Home sick. Played out of position. Fall out with their new managers. Humans are bloody complicated.
When you scout via stats and a guy doesn’t work out, you shrug and point to the same stats as the eye test guys, but hopefully your model has a success rate of better than 50% or what’s the point? As you know from the intro article, the new scouting model that I'm developing backtests quite a bit better than 50%, but guys can still fail to turn into world beaters.
These same problems are also what makes it tough to evaluate model picks. If a guy has one really good year after the model “finds” him, is the pick a success? Two good years? It’s tricky.
Here’s an example: In 09-10, YAPSS (Young Attacking Player Scouting System) said Marko Marin is a prospect teams should be very interested in. Was that pick a success or a failure?
Now Chelsea fans will tell you he failed with them. And yet… He played 1.59 90s in the league while at Chelsea and had a scoring contribution of 1.26 goals and assists per 90. Basically, he couldn’t get on the pitch, but when he did, they scored.
Outside of Chelsea, Marin’s contributed at about a .4 scoring rate wherever he’s gone, including 3G and 9A the year after the model triggered, and he’s a career-long good to great dribbler as well. That has to be a hit, at least statistically.
Football isn’t just stats, but models generally are and need to be evaluated on that basis. The guy I want to talk about today might just be the best statistical prospect in the last half decade (meaning, the entire data set I have access to).
What do you have to do to be labelled “The Best Prospect in Europe?”
You have to be statistically very special.
That’s what Alvaro Morata is.
Name: Alvaro Morata
Age: 21
Position: Center Forward
Team: Real Madrid
Fair Price: £25M
Who should buy him: Every team that needs a forward and can afford him. Actually, throw need out the window. Every team that can afford him. In fact, Real Madrid are dumb to sell him in the first place.
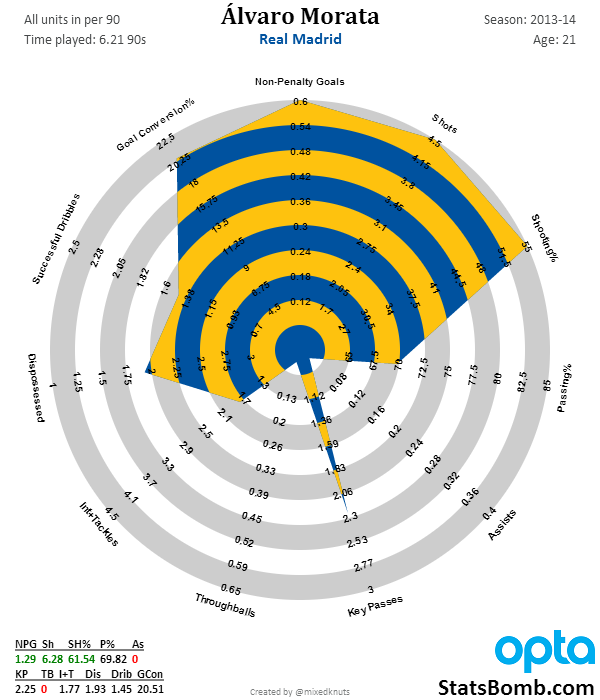
Morata is unreal. 6.2 shots per 90, a non-penalty goal rate of 1.29! 2.25 key passes per 90, 1.45 dribbles… for a guy who is only 21, those stats are absurd.
Check that, for any player in Europe, those stats would be absurd. The list of guys who have shot more than six times per 90 in the last five season is as follows.
Messi. Ronaldo. Wayne Rooney. Mario Balotelli.
So why does Ben (or anyone sensible, really) have reservations? There are a number of good reasons.
- Morata played on Real Madrid, one of the most talented attacking teams in the world. If/when he moves away from there, those stats will fall off because his teammates are unlikely to be as good.
- Morata only played a little over 6 full games in the league this year, and importantly, most of that time came as a substitute, which we know has a big boost on attacking performance.
Those are large asterisks to statistical performance. So why am I still so high on this kid?
The answer is: because of all the young players in the last five seasons of data from all five big leagues in Europe, Morata looks the best. All the other young players to come from top teams, title-winning clubs, minnows, whatever… no one looks as statistically good as Morata.
In five years of data, Mario Balotelli is the only guy to average more than 6 shots per 90 at 23 or younger. The top two young players in Shots per 90 the last four years looks like this:
2013: Balotelli, Nelson Oliveira
2012: Balotelli, Jovetic
2011: Sturridge, Lewandowski
2010: Darron Gibson (no, I have no idea either), Karim Benzema
It’s a pretty strong indicator that a kid is hugely talented.
And here’s the other important thing – he also passes the eye test. He’s 6’3, has a big frame, dribbles extremely well for a big kid, is surprisingly good at picking out teammates with key passes and he’s fast.
Watch his highlights and you’ll see balance, strength, and shockingly soft feet. He scores with both feet (though primarily his right), and heads the ball into the net regularly. He still hasn’t fully filled out his frame, but he’s far from a waif. He also gave Dani Alves a torrid time out on the wing this year in a Classico.
Statistically, we can try to overcome the small sample size a bit by adding in Morata’s earlier time with Real, which includes previous seasons and his Champions League play. When you do that, you wind up with 12.7 90s played, NPG of .86, Scoring Contribution of 1.1, 4.71 shots per90, 1.96 Key Passes, and 1.73 dribbles. All playing for the A team. That’s still bonkers and would be great for his age at half that. His scoring rate at Real B and for the U18, U21, U23 Spanish National teams has been consistently outstanding.
Step back for a second and consider this question. Take what you know about Daniel Strurridge or Robert Lewandowski now and put them on the open market at age 23 so you have all of their prime years ahead of them. What price do you think teams would pay for their services? £50M? £60M?
Jovetic sold for £23M last summer. Lewandowski probably would have sold for £30M with only a year left on his deal, but Dortmund flatly refused to sell to Bayern and made him see out his contract. He’d be worth £60M otherwise. Real bought Benzema for £31M in 2009 and his young player profile wasn’t this good.
I know Morata only has one year left on his deal, and I know there’s some uncertainty from the sample sizes, but from a statistical perspective, it feels like teams should be thinking about how much they would pay for the next Zlatan or Ronaldo or Lewandowski or Sturridge at age 21.
He really does look that good.
If things go badly, you overpay slightly for an average forward. (There's almost no way he's worse than that.) You can probably sell him off somewhere else two years from now for £12-15M to cut your losses.
If things go as the stats suggest they might, you buy the good version of Fernando Torres, right as he is turning into el Nino.
Stop dithering over a couple million pounds. Buy him outright, plug him into your team for the next decade, and enjoy the ride.