Over the past two seasons, I’ve dedicated the majority of my writing towards Europe's prospects and attempting to figure out makes them tick.
While my previous writing slanted towards player profiles on younger players, the general success rate of youngsters coming out of Ligue 1 was what drew my focus. The past two seasons have been an attempt at expanding coverage of young talents using the same model of analysis to cover the other big 5 leagues along with the Eredivisie. I’ve always found it to be more interesting in focusing at the individual level versus the team as a whole when examining football, which could be reasonably seen as a bit counter-intuitive given that soccer isn’t quite like basketball where a superstar can be such a dominating figure in affecting wins/losses. There’s also a much richer tapestry of public football writing at the team level compared to young talents, so there was a niche to be had in attempting to examine prospects at a deeper level.
Part of the inspiration for focusing on individuals comes from a website that actually has nothing to do with football, but rather a basketball website called The Stepien. The Stepien is a website dedicated to in-depth and nuanced coverage on young basketball prospects in relation to the NBA draft, their chances at being able to make it to the NBA, and what their potential ceiling is as prospects once they get into the league. One of the things I appreciate about them is that they’re able to contextualize the strengths/weaknesses of a prospect in relation to the current trends of the NBA and whether that hinders or elevates their standing, to go along with team fit/optimizing player development. Though public writing on footballing young talents has gotten better at including an examination on team fit, it could still do better at also comparing their skillset to the ever-changing landscape of the sport at the highest level.
Given that the season is over and the summer transfer window is nearing, this seemed like an opportune moment to do a deep reflection on some of the bits that I’ve learned from undertaking this two season journey into young talent evaluation. On the whole, I'm not sure how much value this will have, but I think there’s something to be said about having transparency on my end for future reference when looking at players. To be sure, even with what will be said moving forward, I would be the first to tell you that all of this is merely a fraction of what goes on inside clubs, especially ones that have their ducks in a row. People like myself who do this don’t have access to medical or background personal information on young talents when examining them, which are big parts of the overall picture. More than anything, this should be considered musings from someone on the outside.
As for the actual players being scouted, the image below is a rough list of the young players that I’ve watched some level of match footage over the past two seasons, divided into very broad player archetypes. While I’ve dipped my toe into looking at other positions like centre-back and goalkeepers, it would be disingenuous to try and write in detail on them, especially seeing as there are much more qualified individuals that would be valuable resources in that department (Mark Thompson for centre-backs, Paul Riley and David Preece for goalkeepers).
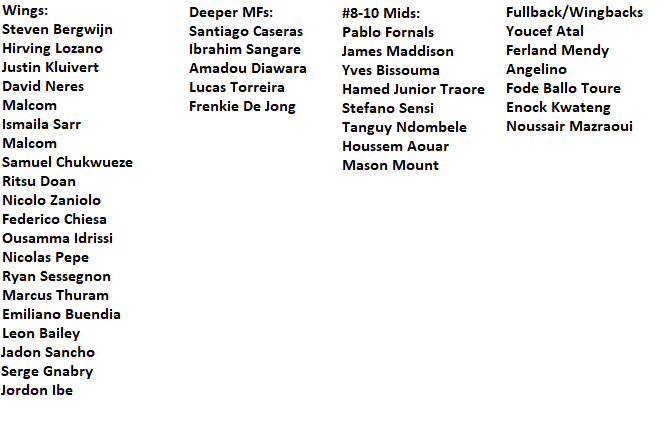
For part 1, we'll be solely looking at wingers. Without further ado...
Wingers:
Of the player archetypes that I’ve looked at, the wide position is definitely the one that I’ve looked at with the most detail. I also think that it’s the position that lends itself best when it comes to using crossover knowledge from basketball.
You can think of wingers in some ways like how analysts contextualize lead initiators in the NBA. The best of the best in basketball are able to shoot in multiple ways from numerous areas on the court (spot-up shooting, shooting off of a live dribble), have the requisite functional athleticism to beat their man with a live dribble, and have vision to make advanced level reads with their passing. Having demerits in either shooting/passing/dribbling pushes you down a rung, and in some instances, hurts your ability to play at the highest level. Certainly with young talents in the NBA, there’s leniency for not seeing that total package right away from the majority of them, but finding enough glimmers of hope for this is the goal. This type of mindset can be transferred quite easily when projecting young wide talents in football.
When people talk about dribbling when it comes to wingers, there can be a lack of analysis outside of “he can beat a man or two”. Certainly, the ability to beat people off the dribble is important, but clarity should be given when describing dribbling aptitude and the process behind it.
There are a multitude of ways for a wide player to execute a 1v1 dribble: cutting inside with their favored foot, walking the sideline by pushing the ball, quick intricate moves in tight spaces, carrying the ball from slightly deeper areas (the Hazard specialty). When it comes to the first one, the importance of gaining access to the halfspace (and beyond) are paramount: greater passing angles, the addition of being a threat to shoot, and even simply the continuation of possession. Finding wide players who are able to do this with some regularity can be such an asset. In sort of the same vein with how shooting is such a bedrock skill in the NBA, dribbling for wide players can be seen in something of a similar light because it is an avenue to unlocking other areas in their game.
Another dribble that some wide players have in their pocket is the ability to push the ball along the wide areas and sprint to receive it themselves while getting past their opponent in the process. This could happen either around the middle third or closer to the penalty box. Though the benefits that come from this play aren’t quite as pronounced as the inside dribble, you can still have better opportunities at delivering low passes/crosses into the box following good execution off the dribble along with getting into the box themselves.
Ismaila Sarr has so far shown enough ability to suggest he'll continue to be really good at this, and his improvement this season has come from his ability to utilize that threat along the sidelines into more playmaking opportunities. Justin Kluivert during his time at Ajax was another example of a winger who could turn his dribbling out wide into something productive near or just inside the wide area of the box.
On the other end of the spectrum, a worry of mine for Oussama Idrissi was that he had issues trying to execute this type of dribble in the Eredivisie (below). While Idrissi was a proficient dribbler on the whole (3.28 dribbles per 90), there were enough instances of him not having that extra gear to make you wonder how he'd do outside of the Eredivisie (it's also fair to point out that his destiny could simply be moving up the ladder and playing for Ajax/PSV, which would alleviate these concerns).
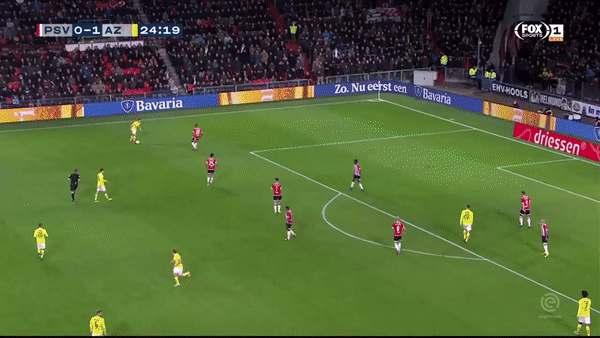
One more note on dribbling skills in isolation: while it's not a death sentence to be extremely one-footed in terms of dribbling acumen, it does put a heightened emphasis on possessing on-ball athleticism. Two test cases for this are David Neres and Samuel Chukwueze. Both of them are on the extreme end of only utilizing their left foot for dribbling and overall on-ball actions, but there's more to believe in with Chukwueze than with Neres given his ball-carrying during transition and greater separation after using feints and sidesteps.
There's also a level of physicality that Chukwueze has that Neres doesn't quite possess, which helps with Chukwueze's dribbling. This isn't to say that I would rank Chukwueze as a better player than Neres, but both players make for an interesting comparison. While dribbling is a foundation skill for wide players and dribbling diversity should be examined, a winger becomes much less interesting if he can't bring much of anything else to the table.
It's all well and good to have the athleticism to rack up dribbling statistics, but if far less good comes out, it just adds up to a shoulder shrug. This is partly why someone like Jordon Ibe hasn't kicked on during his time at Bournemouth because of a lack of definable skills elsewhere post-dribble. It's best to look at wide players who can leverage their dribbling exploits into something greater, either for themselves or others. With regards to the skill intersection of dribbling + shooting, one area that is interesting is being able to create shots off the dribble.
This is admittedly a more niche area given that shots for wide players off the dribble don't make up a large portion of their shot distribution. These shots also tend to be on the lower end of shot quality, but it's still nice to have that shot in your back pocket from time to time when the game mucks down and getting 5-8% shots represents a semi-decent option. Among the many things that Leon Bailey did in 2017/18 that made him a valuable prospect, he definitely would classify as a wide player who could get his own shot.
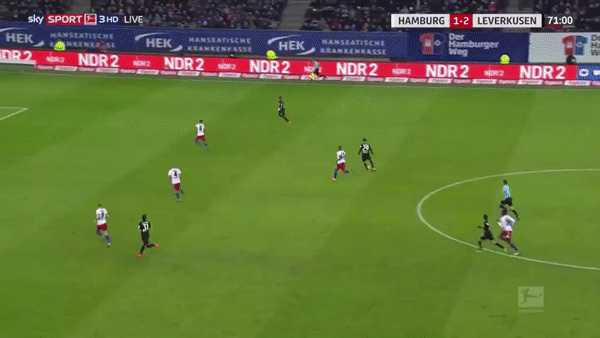
If one was to look at shooting in a more isolated manner, that's where team dynamics become a much bigger factor and how that could affect shot locations. Certainly there's still individual influence that exists with shooting skills. If a wide player is able to have equity as a two-footed shooter or something close to that (Ousmane Dembele for example), that is valuable to have on your squad and makes them less likely to be shaded onto one foot when being defended.
Another skill of shooting when scouting young talents is whether or not there's enough confidence to project them being a good finisher when accounting for shot placement. Though you're overall better off finding wide players who have strong expected goals per 90 rates, because that's more of a repeatable skill over time, finishing skill is still something worth investigating.
Though he no longer qualifies as a young talent, part of the appeal with Nabil Fekir during his younger days was him having a shooting style that would be conducive to outdoing post-shot expected goals models. Serge Gnabry is another test case given his goal tally outpacing pre-shot models in his previous two seasons in Germany, which presented the possibility that he could bring extra value as a plus finisher. It'll be interesting to see if that does turn out to be the case with Gnabry, as he was essentially level with his goal tally this season at Bayern when accounting for placement.
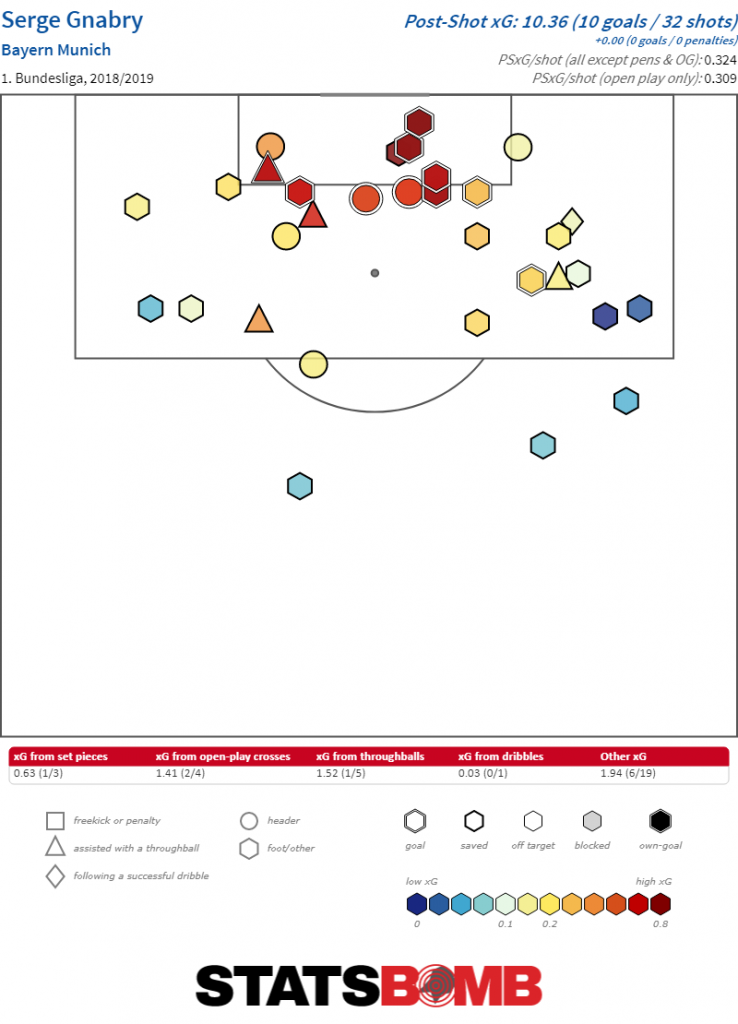
Playmaking responsibilities for wingers have evolved over the past 10-15 years, with full/wing-backs taking up a fair amount of the traditional duties that wingers used to have, one of those being pumping balls into the box from longer distances. This isn't to say that wingers still aren't tasked with lots of playmaking usage, but now you're much more often finding them making shorter ground passes into the box and accumulating open-play key passes in that manner (of course there's an added benefit if wingers can also have some crossing acumen).
There are metrics that when pulled together can give a decent picture at how good a winger is at making plays for others: open-play key passes, expected goals assisted, passes into the box. The more boxes that are ticked, the greater certainty there is. As for how playmaking can translate over film, a good sign is if they're able to have some level of diversity in the way they create their chances. This could be cut-backs, making reverse passes to slip runners at an angle into the wide areas of the box after accessing the halfspace areas, or throughball attempts that split the backline of the defense with different parts of their foot (outside/in/toe poke).
This shows a level of coordination that should instill some confidence that it's translatable across different levels of competition.
An interesting test case for this will be Steven Bergwijn, should he depart from PSV this summer. The Eredivisie has gotten a reputation over the years for not having their talents translate well elsewhere, but Bergwijn has displayed enough diversity with his chance creation over the past two seasons to believe that he'll not be another example of that. He's got near elite touch with his passing in the final third, and he's also able to possess this touch on the move, which is impressive.
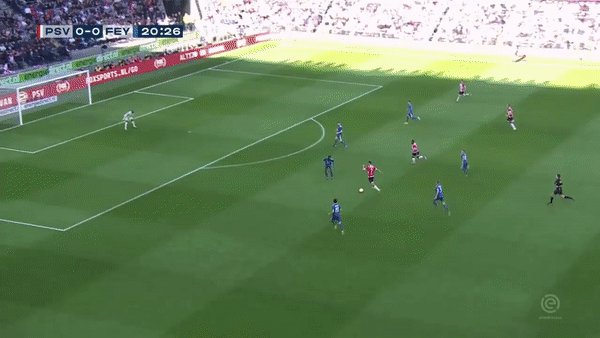
Wingers not only create chances against a set defense, but can also provide value as playmakers during transition opportunities. This could mean that they're the initiators of the counter attack from deeper areas, or ending the transition attack with an incisive pass inside the penalty box. Being a good playmaker during transition involves the combination of decision-making along with on-ball coordination, all the while having to do that at top speed. That is far from being an easy task, which makes what Jadon Sancho has done at Dortmund quite special. He's an absolute terror during Dortmund transitions where he'll receive the ball in space and make either forward passes into the box for open teammate or cutbacks from the right side of the box.
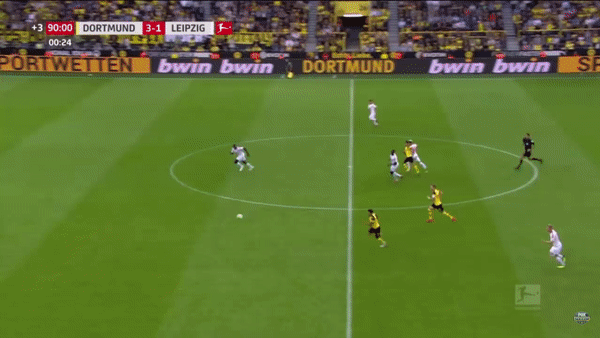
Wingers can also act as playmakers but also have the burden of carrying the ball from deeper areas, particularly if the club is not exactly stacked in collective talent. Malcom's 2017-18 season was filled with these type of moments where he would be utilized as an outlet for transition play, push the ball up the field and still be tasked with making key plays in the final third and penalty box. What was difficult to project was how much this style of play could translate to bigger clubs that dominate play and face more set defenses.
On the negative end, it's a worry when not only a winger doesn't successfully complete difficult passes, but opts to look away and settle for merely recycling the ball. It's one thing to have failed attempts at passes, but to leave stuff off the table in opting for conservatism is a concern. With Nicolo Zaniolo, this was an issue of mine amidst the hype machine that was generating for him during parts of last season. He didn't provide ample value as a passer, which almost made it like Roma were playing with 9 outfield players instead of 10.
Will this linger with Zaniolo the rest of his career, or will it become less of a concern moving forward?
The majority of the discussion has focused on what wingers can do on-ball, but off-ball work is certainly a noteworthy component as well. Wingers who have some questions surrounding their athleticism on-ball can certainly make up some of the lost value with having elite or sub-elite speed + timing with runs. Of course finding wingers who can able perform on/off ball is the dream, but in the absence of that, there's still value to be had by being a speed demon with good positional sense.
Though he is proficient on-ball, Hirving Lozano is damn near special off of it, and Chiesa should project to do well in using his off-ball speed to create shooting opportunities once he gets to a good/great team. For all the worries I've had with David Neres as a prospect (documented here and here), a big reason why I can't get too down on him is because of how dangerous he can be with this part of his game to go along with his passing accumen. Certainly, how much of this skillset he will be able to show outside the Ajax cocoon is a genuine question, but Neres' off-ball work should be able to travel at some level and it's encouraging that he was able to show this part of his game during Ajax's Champions League run.
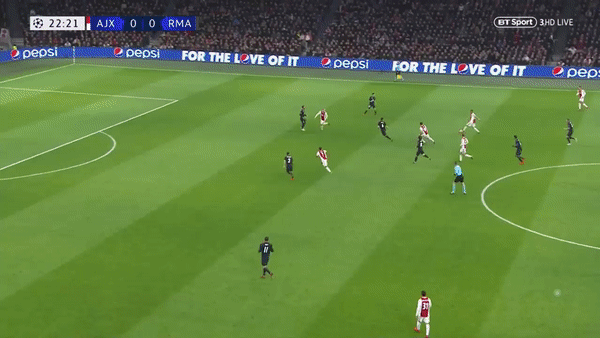
So after all that's been discussed, what can teams try to look for when scouting young wingers? There's no easy answer to this. In a perfect world you would find a prospect who checks off all the boxes, but that's not realistic because at that point, you would be searching for a young Lionel Messi. Dribbling diversity via functional athleticism is a near must, along with the ability to maneuver oneself within the halfspace.
Between looking at post-dribble actions concerning playmaking and shooting, I would lean towards playmaking being more important given the greater likelihood of good-to-great chances being accumulated via post-dribble passes versus individually creating your own shot. Ideally, the winger that's being scouted should have a repertoire of passes into the penalty box that they can make from the final third, but they should at least have the reverse pass into the wide areas of the box as something they can go to when trying to unlock the defense.
There are certainly clubs that would rather find a high-volume shooter from the wide areas rather than a dynamic playmaker, which goes back to team fit and optimization for the scouting club. Before ending part 1, I would be remiss if I didn't touch on perhaps the most intriguing prospect I've come across and one I've talked about quite a bit, Marcus Thuram. If I had to do a big board/ranking of wingers based on current talent level + future upside (something that's done all the time with American sports when looking at young talents), he'd probably be further down the list, but I still find him to be a fascinating player and something of an unknown despite having played over 4000 minutes in Ligue 1 over the past two seasons.
Part of that is due to playing for a small club like Guingamp, while there's also the wonder on whether he'll continue to be a wide player or shift towards more of a central role. The reason why I'm slightly more in favor of letting him continue to start from a wider position for the near future is his high level of functional athleticism to beat people off the dribble using his unique combination of size and speed, in particular his gift for covering ample ground with his first step and his usage of his off-arm to keep opponents away from the ball.
As well, he's shown just enough glimpses of playmaking equity that if I was running a mid/high level club, it would make me want to continue to see just how much he could grow in that part of his game as a winger when surrounded with better overall talent. It's not a situation where there's absolutely nothing to work with his actions post-dribble, though it's fair to wonder just how much room for improvement exists with Thuram's passing.
There's the real chance that should he play on a top 4-6 clubs in a big five league, he would be more of a utility player than a major contributor, but it isn't entirely unreasonable to think that he could hit his higher end outcomes and become a prominent player for notable European clubs if his passing really becomes a strong suit. With Guingamp's relegation to Ligue 2, Thuram should be able to be had at a fairly cheap rate and I would try to get him as a lottery ticket with the knowledge that weren't he not to appreciably improve over time and remain at more of his floor, it wouldn't be too much of a disappointment given transfer fee and wages.
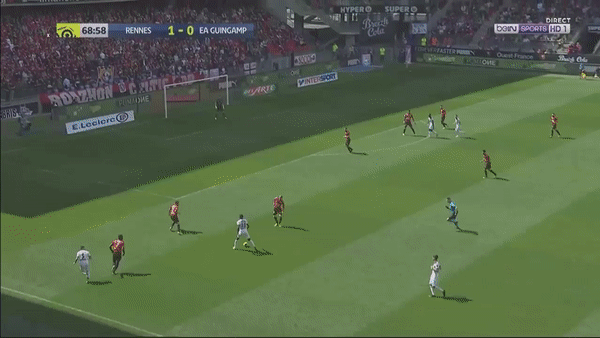
And that's all for wingers. Next week in part two, we'll be looking at midfielders and fullbacks.